Use Google Earth Engine in RStudio with reticulate
A short tutorial on how to use Google Earth Engine inside RStudio with the GEE Python API and reticulate
- the R interface to Python.
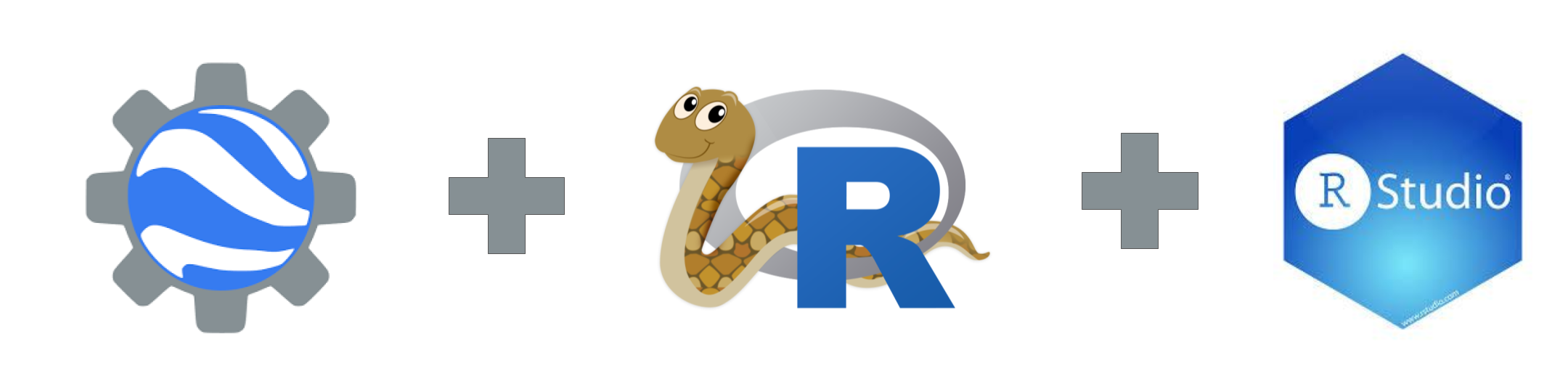
reticulate
.
reticulate
allows us to combine Python and R code in RStudio. So, when values are returned from Python to R they are converted back to R types.
Setup
Where can you get the Earth Engine Python API? Well, there are many installation options, here I use the conda option. The workflow is from Tyler Erickson’s Earth Engine Python API - breakout session from the Geo4Good summit 2019.
Terminal
Open your Terminal and write (check the conda cheat sheet for Windows & Mac differences):
### Terminal
conda create --name gee-demo # Create a conda environment
conda activate gee-demo # Activate the environment
conda install -c conda-forge earthengine-api # Install the Earth Engine Python API
earthengine authenticate # Authenticate your access with the command line tool
conda install pandas
conda install numpy
In RStudio
In RStudio, install the reticulate
package and apply the use_condaenv()
function, which enables you to specify which version of Python in the conda
environment you want to use.
### R
install.packages(reticulate)
library(reticulate)
use_condaenv("gee-demo", conda = "auto",required = TRUE)
Import the Earth Engine API (ee) and run the ee$Initialize
function to trigger the authentication.
### R
ee = import("ee") # Import the Earth Engine library
ee$Initialize() # Trigger the authentication
np = import("numpy") # Import Numpy
pd = import("pandas") # Import Pandas
Get Sentinel-2 data from Earth Engine
Let’s assume we are interested in a high resolution, cloud free summer scene from a urban green area in Berlin, Germany. Here is the workflow to get the data into R.
### R
geometry = ee$Geometry$Point(13.481643640792527,52.48959983479137);
S2 = ee$ImageCollection("COPERNICUS/S2_SR")
# select images between May and August of 2019
S2 = S2$filterDate(ee$Date('2019-05-01'), ee$Date('2019-08-01'))$filterBounds(geometry)
Check the number of images in the Image Collection
### R
nbrImages = S2$size()$getInfo()
nbrImages
# 71
Order all images according to their cloudiness and select the first (least clouds) image. Create a green vegetation layer (NDVI) and convert the ee.Image
to a numpy
array.
### R
S2 = S2$sort("CLOUD_COVERAGE_ASSESSMENT")$first() # select the least cloudy image
S2 = S2$select(list("B2", "B3", "B4", "B8"))
ndvi = S2$normalizedDifference(c('B8', 'B4')) # get the NDVI as new layer
ndvi = ndvi$multiply(1000)$clip(geometry$buffer(500))$rename("ndvi") # clip to the park area
latlng = ee$Image$pixelLonLat()$addBands(S2)$addBands(ndvi) # get selected bands and ndvi
latlng = latlng$reduceRegion(reducer = ee$Reducer$toList(),
geometry = geometry$buffer(500),
maxPixels = 1e8,
scale = 10)
lats = np$array((ee$Array(latlng$get("latitude"))$getInfo())) # convert lat lon values to numpy array
lngs = np$array((ee$Array(latlng$get("longitude"))$getInfo()))
ndvi_values = np$array((ee$Array(latlng$get("ndvi"))$getInfo())) # convert band values to numpy array
B2_values = np$array((ee$Array(latlng$get("B2"))$getInfo()))
B3_values = np$array((ee$Array(latlng$get("B3"))$getInfo()))
B4_values = np$array((ee$Array(latlng$get("B4"))$getInfo()))
B8_values = np$array((ee$Array(latlng$get("B8"))$getInfo()))
Convert numpy array to raster
Convert the numpy
array (Python) to a data.frame
® and raster
® data types.
### R
df <- data.frame(x = lngs, y = lats, ndvi = ndvi_values, B2 = B2_values,
B3 = B3_values, B4 = B4_values, B8 = B8_values)
library(raster)
XYZ <- rasterFromXYZ(df)
Plot False & True Colour
Once we have the raster stack, we can start to prepare a false colour or true colour plot based on exported layers.
### R
plotRGB(XYZ, 5, 4, 3, interpolate = TRUE)
plotRGB(XYZ, 4, 3, 2, interpolate = TRUE, stretch = "lin")
Rayshader
Last but not least I wanted to try the new rayshader
library and render a 3D NDVI map with depth of field.
### R
library(rayshader)
ndvi_m = raster_to_matrix(XYZ$ndvi)
ndvi_m %>%
sphere_shade(texture = "desert") %>%
add_water(detect_water(ndvi_m), color = "lightblue") %>%
add_shadow(ray_shade(ndvi_m, zscale = 3), 0.5) %>%
add_shadow(ambient_shade(ndvi_m), 0.5) %>%
plot_3d(ndvi_m, zscale = 10, fov = 30, theta = -225, phi = 25, windowsize = c(1000, 800), zoom = 0.3)
render_depth(focus = 0.6, focallength = 200, clear = TRUE)
render_snapshot(clear = TRUE)
Session Info
Here is my version information about R, the OS (Mac) and attached or loaded packages for comparison.
### R
sessionInfo()
## R version 3.4.3 (2017-11-30)
## Platform: x86_64-apple-darwin15.6.0 (64-bit)
## Running under: macOS High Sierra 10.13.6
##
## Matrix products: default
## BLAS: /Library/Frameworks/R.framework/Versions/3.4/Resources/lib/libRblas.0.dylib
## LAPACK: /Library/Frameworks/R.framework/Versions/3.4/Resources/lib/libRlapack.dylib
##
## locale:
## [1] en_US.UTF-8/en_US.UTF-8/en_US.UTF-8/C/en_US.UTF-8/en_US.UTF-8
##
## attached base packages:
## [1] stats graphics grDevices utils datasets methods base
##
## loaded via a namespace (and not attached):
## [1] compiler_3.4.3 magrittr_1.5 tools_3.4.3 htmltools_0.3.6
## [5] yaml_2.2.0 Rcpp_1.0.1 stringi_1.3.1 rmarkdown_1.12
## [9] knitr_1.22 stringr_1.4.0 xfun_0.8 digest_0.6.18
## [13] evaluate_0.13
Bottom Line
If you have any questions, suggestions or spotted a mistake, please use the comment function at the bottom of this page.
Previous blog posts are available within the blog archive. Feel free to connect or follow me on Twitter - @Mixed_Pixels.